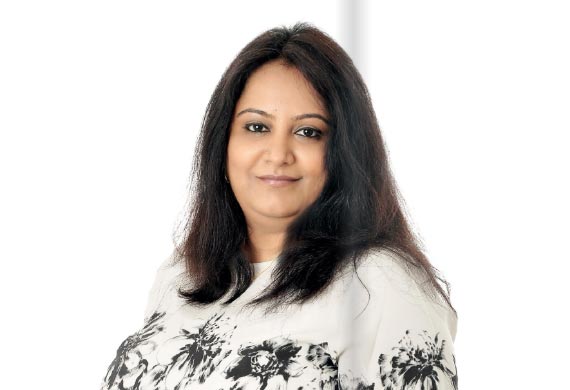
Why Traditional Early Warning Systems Have Failed. ML And Signal-Driven Systems Can Solve The NPA Problem
By: Meghna Suryakumar, Co-Founder and CEO, Crediwatch
In addition to topnotch expertise in New Ventures, AI, Deep Technologies, AML, KYC, Financial Inclusion, Risk Intelligence and Amplified Intelligence for Businesses, she is leading the firm with her business excellent acumen.
Credit lending in the past has favoured those with affluent backgrounds, leaving underprivileged customers without any way to obtain loans from the formal sector. This creates a vicious cycle because, without a loan, they cannot build a credit history, which again affects their ability to get loans. Lenders generally assess a borrower’s creditworthiness based on their previous repayment data, and therefore, the underbanked are often neglected. MSMEs, in particular, find it extremely challenging to avail credit from banks, NFBCs and other established financial institutions. According to the World Bank, the total credit gap in the MSME sector in India stands at $380 billion, which is a reminder that traditional data-based credit scoring methods have failed to serve to the small businesses in the country.
Since traditional credit scoring relies on existing infrastructure and data inputs like financial assets and collateral, it restricts access to formal credit. On the other hand, this method is also proven inadequate to reduce false positives and bad loans. That is because traditional data offers very little information about a borrower’s capacity, character and willingness to repay the loan. Given these limitations, lenders are now moving away from traditional credit scoring to an alternative datadriven credit evaluation system that analyzes both behavioural and social information from various online sources to determine the creditworthiness of loan applicants.
“The Indian banking sector is reeling under the stress of bad loans. Government statistics reveal that India’s 42 scheduled commercial banks (SCBS) collectively wrote off bad loans worth RS 2.12 trillion in 2018-19”
What is alternative data?
Alternative data refers to any information that can be used to establish the creditworthiness of a borrower in a non-traditional manner. Alternative data goes beyond the information about borrower’s past history of borrowing and repayments, and assesses the businesses’ interaction with regulators, business partners and legal parties. It has been observed that such seemingly arbitrary information can accurately indicate one’s trustworthiness. Alternative data-based credit scores can play a crucial role in gauging the risk of default, especially in emerging economies like India where the lack of reliable data on an individual’s past credit behaviour makes a vast majority of the population financially invisible. Given the growing number of internet users in the country, lenders can easily access large volumes of alternative data with user consent.
How can alternative credit scoring help lenders?
The Indian banking sector is reeling under the stress of bad loans. Government statistics reveal that India’s 42 scheduled commercial banks (SCBs) collectively wrote off bad loans worth Rs 2.12 trillion in 2018-19. While private banks have also faced the challenge of loan defaults and NPAs, the bad loan crisis is more prominent among public sector banks despite government efforts.
Following the Asset Quality Review 2015, the Reserve Bank of India (RBI) mandated the adoption of EWS to as a best practice in detecting and mitigating the risks posed by Red Flagged Accounts (RFAs). However, this move has not been able to help banks keep away from bad loans. Since 2014-15, Indian banks have written off bad loans worth Rs 5.7 trillion. Many reasons have contributed to this, including the fact that traditional Early Warning Systems do not include alternative data for credit risk assessment, thereby leaving many blind spots.
Early Warning Systems (EWS) based on alternative data can avert this crisis by alerting lenders about any suspicious activities early on. New-age Early Warning Systems leverage advanced technologies such as artificial intelligence (AI), machine learning (ML), analytics and data modelling to analyze the data mined from multiple touchpoints and monitor and measure risk.
Alternative data, when combined with traditional data, enables lenders to avoid systematic risks and extend their lending network. While there have been long-going discussions around incorporating non-traditional data into credit scoring, now is the time to build a robust credit evaluation system that can merge alternative data with traditional data to create a win-win situation for lenders as well as borrowers.